About Me
I’m a PhD student at the Stanford Institute for Computational and Mathematical Engineering (ICME), advised by Marco Pavone. I received my B.S. from Caltech in 2018, where I double majored in Mathematics and Business, Economics, & Management, and minored in Control & Dynamical Systems. I have completed research internships with the USRA-NASA Quantum Artificial Intelligence Laboratory and the Amazon Modeling & Optimization team.
Contact: rabrown1 [at] stanford [dot] edu
Research
I am broadly interested in optimization, with various applications including quantum computing, multi-agent control, machine learning, and supply chain management.
My most recent focus involves the design and analysis of hybrid algorithms that leverage the capabilities of the Coherent Ising Machine. This research aims to provide insights for the synergistic co-design of “hardware primitives” and optimization algorithms, and has applications in neural network verification. Additionally, I investigate the integration of classical optimization methods into unconventional computing architectures.
Additional topics I have worked on include distributed optimization for multi-agent systems, and reinforcement learning for supply chain management.
Preprints
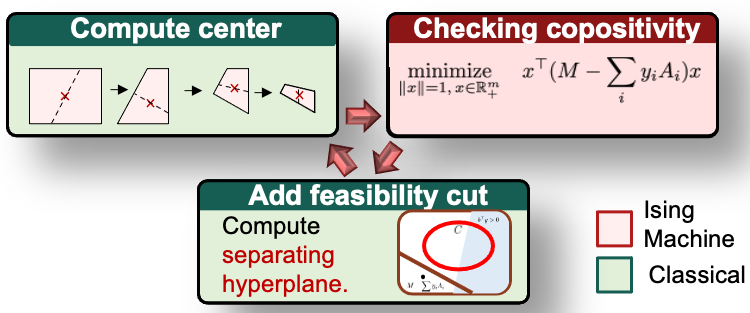
- Under Review - 2023
We integrate Adam and momentume optimizers with the continuous variable Coherent Ising Machine (CV-CIM) dynamical solver. We show that both optimization techniques can improve the convergence speed and sample diversity of the CV-CIM, while Adam improves the stability of the resulting system
Publications
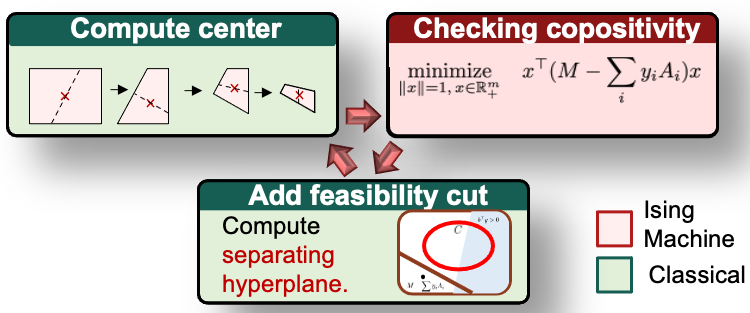
- SIAM Journal of Optimization - 2023
We present a formal analysis of hybrid algorithms in the context of solving mixed-binary quadratic programs (MBQP) via Ising solvers. We leverage copositive optimization and cutting-plane algorithms to derive an algorithm that provable shifts complexity onto the subroutine handled by the Ising solver.
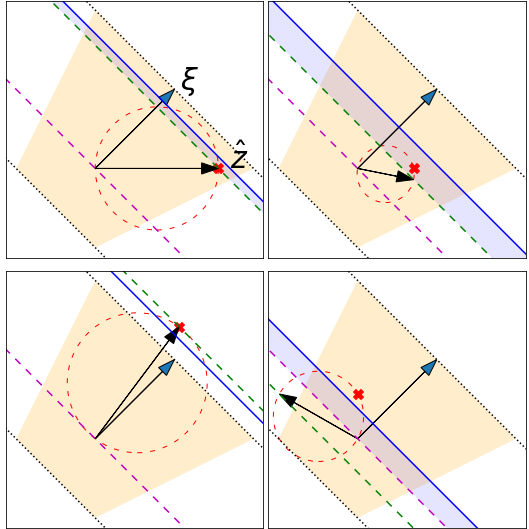
- Int. Conf. on Artificial Intelligence and Statistics - 2022
We unify several SDP relaxations for ReLU neural network verification by providing an exact convex formulation as a completely-positive program. This provides a path for relaxations that systematically trade off tightness and efficiency.
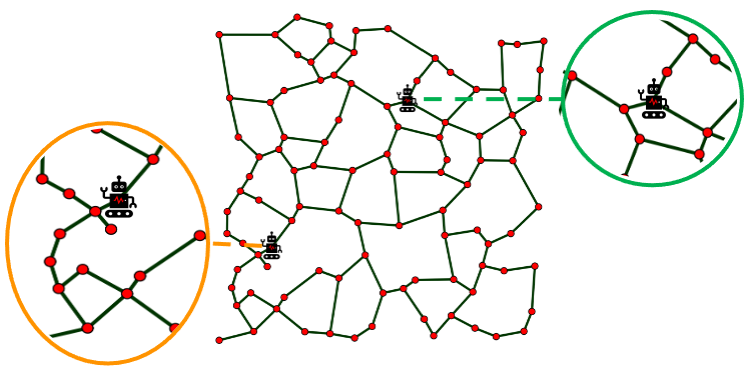
- IEEE Transactions on Control of Network Systems - 2021
We extend our prior work by providing tighter bounds on the locality of problems through the conjugate-gradient algorithm, allowing the decay results to be applied to all linearly constrained strongly convex optimization problems.
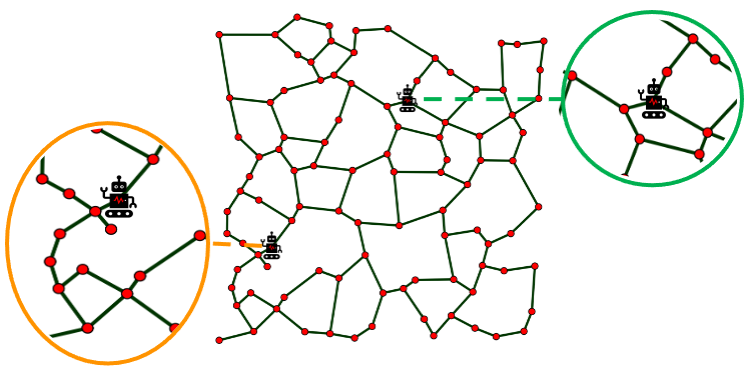
- European Control Conference (Best Student Paper Award Finalist) - 2020
We develop a rigorous measure of “locality” that relates the structural properties of a linearly-constrained convex optimization problem to the amount of information that agents should exchange to compute an arbitrarily high-quality approximation of its solution. We leverage the notion of locality to develop a locality-aware distributed optimization algorithm.